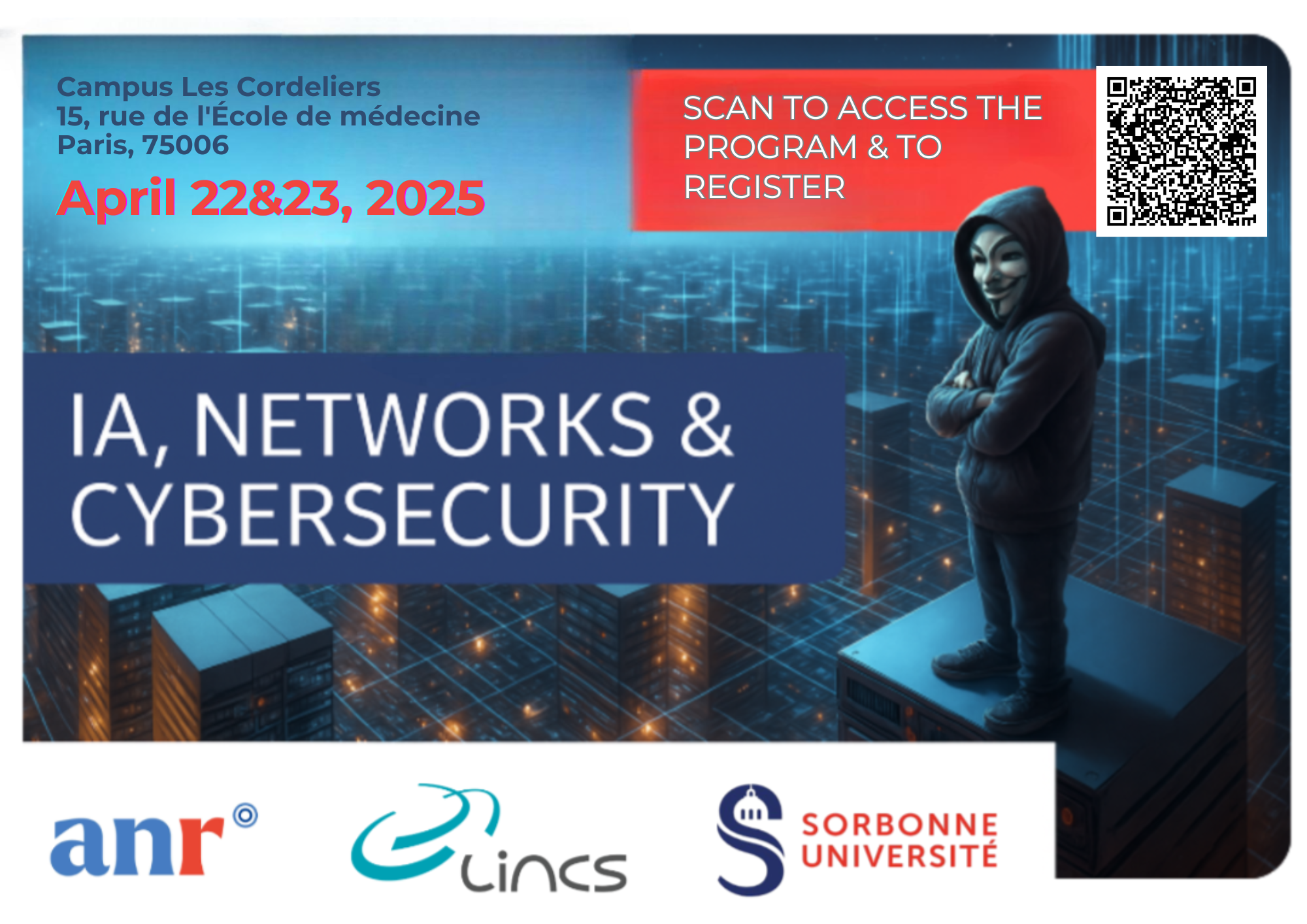
We are pleased to announce the upcoming Joint LINCS / Sorbonne University / ANR – Grifin Workshop on AI, Networks & Cybersecurity, which will take place on April 22 & 23, 2025, in Sorbonne University, Les Cordeliers campus, 15 rue de l’École-de-médecine, 75006 Paris.
Registration
- Registration is mandatory.
- Are you a PhD Student or a Postdoc and want to be part of it? You can submit your poster and give an elevator pitch! Fill in the blanks.
Speakers
April 22 – Day 1
- 9h30-10h15: Accueil/Café
- 10h15-10h30: Introduction (presentation of LINCS, GRIFIN, and program)
- 10h30-11h00: “How dataset quality shapes resistance to data poisoning”, by Katarzyna Wasielewska
- 11h00-11h30: “Network Data Augmentation Through Protocol-Constrained Traffic Generation” , by Francesco Bronzino
- 11h30-12h30: “AI for the Detection and Mitigation of Cyberattacks” , by Erol Gelenbe
- 12h30-14h00: Lunch
- 14h00-14h30: Elevator Pitch
- “Cybersecurity impact of AI Optimization in B5G networks”, by Alex Pierron
- “Privacy Benchmarking of IDS with FREIDA”, by Solayman Ayoubi
- “MetaLore: Learning to Orchestrate Communication and Computational Resources for Metaverse Synchronization” by Ohri Elif
- “Studying Gossip Learning” by Alexandre Pham
- 14h30-15h15: Poster around coffee
- 15h15-16h15: “Collaborative Learning attacks and defenses”, by Alice Héliou
- 16h15-17h15: “A Small Tutorial on Byzantine-Robustness – Federated Learning with adversarial nodes”, by Rafael Pinot
April 23 – Day 2
- 09h30-10h30: “Securing the Future: Understanding Attacks on AI-Driven Network Management”, by Valeria Loscri
- 10h30-11h00: Pause café
- 11h00-11h30: “Can we still learn something from Darknet traffic?”, by Jérôme François
- 11h30-12h00: “Automating security management for the cyberspace”, by Rémi Badonnel
- 12h00-12h30: “Advanced Network Fuzzing for Networked System Testing” , by W. Mallouli
- 12h30-14h00: Lunch
- 14h00-15h00: Elevator pitches
- “Improving anonymous secure communications on the Internet”, by Guillaume Nibert
- “HEAL: Resilient and Self-* Hub-based Learning” by Mohamed Amine Legheraba
- “DDoS Mitigation while Preserving QoS: A Deep Reinforcement Learning-Based Approach” by Shurok Khozam
- “A genetic algorithm approach to flight optimization”, by Massinissa Tighilt
- 14h30-15h30: Poster around coffee
- 15h30-16h00: “Data quality: the key to automation”, by José Camacho
- 16h00-16h30: “Managing the cloud-to-edge continuum under uncertainty via AI methods with performance guarantees” by Andrea Araldo
- 16:30-16:45: Conclusion
Talks
Andrea Araldo (Télécom Sud Paris)
“Managing the cloud-to-edge continuum under uncertainty via AI methods with performance guarantees”
There is a long tradition of network management methods based on a precise model of the network and of the load. However, in practical situations it is impossible to build such a model, mainly because the load is uncertain and not known in advance. AI methods can overcome this model/reality gap, by continuously adjusting decisions based on streams of monitoring observations.
In this talk, I will show how we applied AI to manage the cloud-to-edge continuum, focusing on the following decisions: pricing, placement of multiple “versions” of machine learning models, resource allocation. The methods we applied are Hidden Parameter Markov Decision Processes, Model-Based QLearning, Online Learning. I will show that, despite the uncertainty on the input load, we are able to provide analytic guarantees on the worst-case performance or on the average performance. Such guarantees are important to foster the applicability of AI algorithms, which is often hindered by their black-box nature.
Rémi Badonnel (Loria)
“Automating security management for the cyberspace”
The Internet has become a great integration platform capable of efficiently interconnecting billions of entities, from simple sensors to large data centers. This platform provides access to multiple hardware and virtualized resources (servers, networking, storage, applications, connected objects) ranging from cloud computing to Internet-of-Things infrastructures. From these resources that may be hosted and distributed amongst different providers and tenants, the building and operation of complex and value-added networked systems is enabled. These systems are however exposed to a large variety of security attacks, that are also gaining in sophistication and coordination.
In that context, we investigate challenges about automating security management for the cyber-space, through the development of new monitoring and configuration solutions tailored to these systems.
Francesco Bronzino (ENS Lyon)
“Network Data Augmentation Through Protocol-Constrained Traffic Generation”
Traffic Generation Datasets of labeled network traces are essential for a multitude of machine learning (ML) tasks in networking, yet their availability is hindered by privacy and maintenance concerns, such as data staleness. To overcome this limitation, synthetic network traces can often augment existing datasets. Unfortunately, current synthetic trace generation methods, which typically produce only aggregated flow statistics or a few selected packet attributes, do not always suffice, especially when model training relies on having features that are only available from packet traces. This shortfall manifests in sub-optimal performance on ML tasks when employed for data augmentation.
In this talk, we discuss our ongoing work on developing generative techniques to augment network datasets for a variety of tasks, from traffic classification to QoE measurement. First, we present NetDiffusion, a tool that uses a finely-tuned, controlled variant of a Stable Diffusion model to generate synthetic network traffic that is high fidelity and conforms to protocol specifications. Our evaluation demonstrates that packet captures generated from NetDiffusion can achieve higher statistical similarity to real data and improved ML model performance than current state-of-the-art approaches (e.g., GAN-based approaches). Second, we discuss how this approach is more suited to support common network analysis tasks, as well as our ongoing efforts solving NetDiffusion’s limitations.
José Camacho (University of Granada)
“Data quality: the key to automation”
Data quality is a central topic often neglected in Data Science. With the current interest in AI and Deep Learning, some data scientists believe that creating a successful data analysis pipeline is simply a matter of finding a suitable AI model, disregarding the possibility that the data itself may be poor. This view conflicts with the old Data Science mantra, “Garbage In, Garbage Out,” which dates back to the origins of computational science. Can you predict when you will suffer from cancer, if at all, based on your eye color? Probably not. Can you detect a cybersecurity attack only from traffic traces? Well, maybe, but that might not be sufficient.
In my experience, many data pipelines succeed only after proper experimental design (that controls data generation), data visualization and understanding, filtering, cleaning, transformation, and data fusion from complementary sources. In my experience, again, 75% of a data scientist’s traditional work is often devoted to data preparation, which is quite complicated to automate.
In this talk, I will discuss some of the pitfalls we face when designing an AI pipeline, drawing examples from areas other than autonomic networks, and I will make the association with challenges for AI automation in network management.
Jérôme François (University of Luxembourg)
“Can we still learn something from Darknet traffic?”
Darknet allows to collect supposed malicious traffic by exposing entire IP subnetworks into the wild. They have been used for more than two decades for monitoring large threats over Internet such as DDoS, botnets or probing activities. Their passive nature limits the knowledge that can be gathered beyond these large phenomena.
Using the Darknet of the High Security Lab in Inria Nancy, we have performed several analysis over the years showing that Darknet can still be used to anticipate some threats or extract relevant knowledge to perform traffic analysis in other networks. We’ll briefly these different approaches and showing limitations and remaining opportunities.
Erol Gelenbe (Institute of Theoretical & Applied Informatics, Polish Academy of Sciences, King’s College London & CNRS I3S, Université Côte d’Azur, Nice, France)
AI for the Detection and Mitigation of Cyberattacks
Gateway Servers for the Internet of Things are used in critical application areas such as industrial IoT, the Internet of Vehicles and health monitoring. Thus they must meet stringent Security and Quality of Service (QoS) requirements, offering cyber-attack protection with fast response and minimal loss of benign data. Therefore, protecting these systems with effective traffic shaping, accurate Attack Detection (AD) and Mitigation mechanisms is vital.
We will first demonstrate online and federated learning techniques that accurately detect attacks. Measurements of packet floods that convey a cyber-attack will be shown to impair the QoS at the Gateways and impede their capability to carry out AD. Using Queueing Theory, and experimental measurements, we show that the novel traffic shaping method QDTP ensures that a Gateway can allow AD to operate promptly during an attack. A new Adaptive Attack Mitigation (AAM) system is then introduced to sample the incoming packet stream, determine whether an attack is ongoing, and dynamically drop batches of packets at the input to reduce the effects of the attack, and minimize the AD overhead and the cost of lost benign packets.
Alice Héliou (Thales)
“Collaborative Learning attacks and defenses”
Collaborative learning allows to work together to train better models without directly exchanging data. Although very powerful, applying secure, robust, and ethical machine learning approaches in a collaborative setting proves to be more complex than expected.
This talk will present our past, current, and future work on attacks and defenses applied to collaborative learning.
Valeria Loscri (Inria)
“Securing the Future: Understanding Attacks on AI-Driven Network Management”
In the context of network management, the integration of Artificial Intelligence (AI) and Machine Learning (ML) is gaining momentum, permitting automation and optimization. However, integrating AI/ML into network management, changes the security landscape. From one side, security can be improved, by enhancing threat and anomaly detection, by enabling a more rapid response to threats. Moreover, vulnerabilities can be predicted and patches can be considered to avoid the exploitation of such vulnerabilities to convey impacting attacks. From another side, AI/ML-based approaches are prone to different types of attacks, ranging from adversarial attacks, data privacy and confidentiality and model drift. These points need to be carefully considered and to mitigate security risks some key aspects related to secure AI/ML training and deployment and adversarial defense mechanism need to be explicitly considered.
In this talk, we will review the benefits of AI/ML in network management as well as their dark side when employed in network management.
Wissam Mallouli (Montimage)
“Advanced Network Fuzzing for Networked System Testing”
This session will provide both theoretical insights and practical demonstrations using the Montimage Network Fuzzer, an open-source tool designed to enhance automated testing.
Rafael Pinot (Sorbonne University)
“A Small Tutorial on Byzantine-Robustness – Federated Learning with adversarial nodes”
The vast amount of data collected every day, combined with the increasing complexity of machine learning models, has led to the emergence of distributed learning schemes. In the now classical Federated learning architecture, the learning procedure consists of multiple data owners (or clients) collaborating to build a global model with the help of a central entity (the server), typically using a distributed variant of SGD. Nevertheless, this algorithm is vulnerable to ” misbehaving ” clients that could (either intentionally or inadvertently) sabotage the learning by sending arbitrarily bad gradients to the server. These clients are commonly referred to as Byzantine and can model very versatile behaviors going from crashing machines in a datacenter to colluding bots attempting to biase the outcome of a poll on the internet.
The purpose of this talk is to present a small introduction the emerging topic of Byzantine Robustness. Essentially, the goal is to enhance distributed optimization algorithms, such as distributed SGD, in a way that guarantees convergence despite the presence of some Byzantine clients. We will take the time to present the setting and review some recent results as well as open problems in the community.
Katarzyna Wasielewska (University of Granada)
“How dataset quality shapes resistance to data poisoning”
Data poisoning attacks can introduce false data into the training dataset, causing ML models to make incorrect and misleading decisions. These types of attacks exploit weaknesses in datasets.
In this talk, we will try to answer the question of whether dataset quality is the first line of defense against data poisoning in AI systems.
Elevator Pitch Session
Solayman Ayoubi (Sorbonne University)
“Privacy Benchmarking of IDS with FREIDA”
Ohri Elif (Sorbonne University)
“MetaLore: Learning to Orchestrate Communication and Computational Resources for Metaverse Synchronization”
Shurok Khozam (Telecom SudParis)
“HEAL: DDoS Mitigation while Preserving QoS: A Deep Reinforcement Learning-Based Approach”
Mohamed Amine Legheraba (Sorbonne University)
“HEAL: Resilient and Self-* Hub-based Learning”
Guillaume Nibert (Sorbonne University)
“Improving anonymous secure communications on the Internet”
Alexandre Pham (Sorbonne University)
“Studying Gossip Learning”
Alex Pierron (Telecom SudParis)
“Cybersecurity impact of AI Optimization in B5G networks”
Massinissa Tighilt (Sorbonne University)
“A genetic algorithm approach to flight optimization”